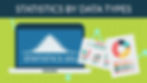
Data plays a significant role in the field of data science. Raw data is put into enormous experiments to generate meaningful insights that drive many business goals. Understanding different data types in statistics will take you one step closer to picking the data type that matches your business requirements. The data type knowledge will help you accurately apply statistical measurement to raw data and conclude meaningful insights. You must be able to identify the data type you are working with if you want to select the apt visualization method.
Explained below are the different data types in statistics that you must know for performing proper exploratory data analysis (EDA).

Qualitative data can be further divided into two subtypes:
Nominal Data: This type of qualitative data represents categories without any inherent order or ranking. Examples include:
Types of animals (e.g., cat, dog, bird)
Marital status (e.g., single, married, divorced)
Political affiliation (e.g., Democrat, Republican, Independent)
Ordinal Data: This type of qualitative data represents categories with a specific order or ranking. While the categories have a relative ranking, the magnitude between categories may not be precisely quantifiable. Examples include:
Educational levels (e.g., elementary, high school, college)
Rating scales (e.g., poor, fair, good, excellent)
Likert scale responses (e.g., strongly agree, agree, neutral, disagree, strongly disagree)
Qualitative data is often collected through methods such as interviews, observations, or open-ended survey questions. Analyzing qualitative data typically involves techniques such as thematic analysis, content analysis, or grounded theory, focusing on identifying patterns, themes, and insights from the data.
Quantitative data, as opposed to qualitative data, is numerical in nature and represents quantities or measurements. It can be analyzed using mathematical and statistical methods to derive meaningful insights and draw conclusions. Quantitative data is objective and can be subjected to various mathematical operations and statistical analyses.
Quantitative data can be further categorized into two main types:
Continuous Data: Continuous data represents measurements that can take any value within a specific range. It can be divided into smaller and smaller units, making it possible to have fractions or decimal values. Examples include:
Height of individuals (e.g., 165.5 cm, 180.2 cm)
Temperature readings (e.g., 24.7°C, 35.2°C)
Time taken to complete a task (e.g., 10.3 seconds, 45.6 seconds)
Discrete Data: Discrete data represents measurements that can only take specific, separate values. It often involves counting or whole numbers. Examples include:
Number of siblings in a family (e.g., 1, 3, 5)
Number of items sold (e.g., 10, 25, 50)
Customer ratings on a scale of 1 to 5 (e.g., 3, 4, 5)
Quantitative data is commonly collected through methods such as surveys, experiments, or direct measurements. It allows for various statistical analyses, such as descriptive statistics (e.g., mean, median, standard deviation), inferential statistics (e.g., hypothesis testing, regression analysis), and data visualization techniques (e.g., histograms, scatter plots) to explore relationships, patterns, and trends in the data.